Unlock 2.5% – 4% global revenue growth.
Making things easy online has never been more important. With 2019’s shelf-scanners now scroll-hover-clicking and digital spend still 32% above pre-pandemic levels*, legions of new-to-digital customers are demanding the ease of IRL when they shop online.
And now with simpler-than-ever product recommendations, there’s no longer any excuse. Find out what you need to get right and who to hire to unlock 2-5% – 4% global incremental revenue growth*.
In this article we will cover:
- The power of Product Recommendations
- 6 components to get right, each including:
- Complexity scores
- Expected timelines
- Potential value add
- Who to hire to achieve all of this
Product Recommendations, save us from this hell
In 2019 you were drawn in by the window display before strolling inside; casting around at the models; dancing from jeans to shoes and, with your side-eyes, building an impression of a brand, its products and its identity. You left with your hands full and, occasionally, formed a meaningful & enduring connection.
Today you are slumped on the sofa. There is no dancing, no chance encounters and a ubiquitous lack of joy. If you were one of the millions* drawn online during the pandemic, you’d be having even less fun.
It’s quick, but it’s crap.
OK it’s not that bad, but most will agree there is generally less joy behind the screen. This is seen as unfortunate, but an acceptable trade-off for ecommerce’s breathtaking convenience.
But it doesn’t have to be.
You can still dance from jeans to shoes.
You can still look at a shoe, with your side-eyes frolicing merrily.
Well implemented product recommendations bring back the window display, the other side of the store, the ready-made outfits. The online convenience with the random & unpredictable joy of the physical.
Personalised product recommendations are something quite different. A store where everything’s your size, where we know you have too many t-shirts, we know what goes with these trousers and we know what you don’t know (this t-shirt is different, you’re going to love it!).
And there’s no excuse. Tech sophistication continues to evolve rapidly and today there are vendors queueing up to do it all for you. The most transformative of these can initially be tricky, but it’s always easy when you know how. If you decide today to give it a go, here’s what you need to get right.
What are these 6 crucial components then!?
1) Product Library: sounds obvious, but this can be fun.
First, what is a product? An article, a form, a piece of content, a dress, a semiconductor.
Then what do we know about it? A good tool will allow you to augment back-end product data with info from the page including real-time time-sensitive updates like stock level.
Getting it done:
6/10
tricky
2
weeks
Getting it right:
8/10
tricky
4
weeks
You need: developer with ~3yrs recommendations-tool-specific experience & TMS developer with experience of both your tag management system & this same recommendations-tool.
The hard part
- Data architecture (translating back-end & datalayer product schemas into recommendations-tool product feed data schema)
- Market-specific environment mapping need to be well thought through

Complexities such as large, multi-market product libraries & quality control for low-traffic products

Outcome: This is a big part of what you're paying for and by getting it right, you're ready to scale to 2.5% - 4% revenue growth that will come later.
“you’re ready to scale the 2.5% – 4% revenue growth that will come later”
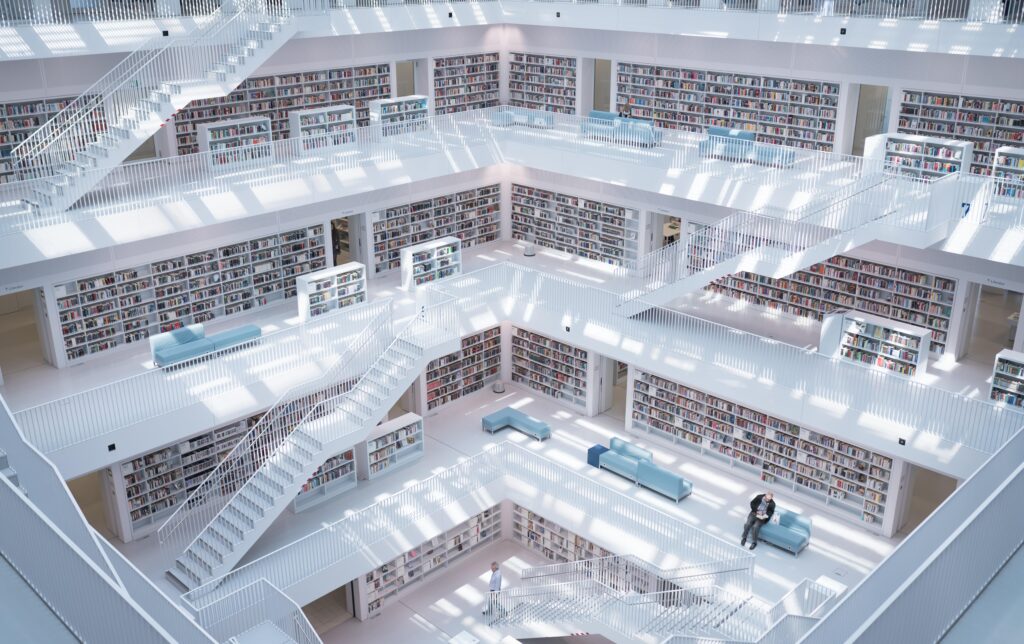
2) Product Metadata: If your product library is the bricks & mortar, product metadata is the books on the shelves.
You must be 100% sure customers will see the right products in the right market, with the correct metadata.
A single error here at best will damage trust and at worst could present customers with dangerous misinformation.
Getting it done:
4/10
tricky
1
week
Getting it right:
8/10
tricky
3
weeks
You need: data architect with ~3yrs recommendations-tool-specific experience & TMS developer with experience of the recommendations-tool & your tag management system.
The hard part
- Attributes need to be identified & captured before you need them, so you need either an experienced data architect or CRO/ Personalisation roadmap specialists working very closely with your recommendations-tool-specific developers

Standardising & validating data cross-page & cross-market demand careful attention, while complexities such as currencies & translation localisations are best owned by developers with in-depth tech-specific experience.

Outcome: ubiquitous and reliable metadata opens up entirely new use cases beyond the threshold of behavioural-only modelling. This is new revenue and includes services customers expect to see, such as affinity-based recommendations and those identifying commonalities across myriad different product-specific data points. DMPG clients make 10-30% of program revenue from recommendations dependent on ubiquitous & reliable product metadata.
“DMPG clients make 10-30% of program revenue from recommendations dependent on ubiquitous & reliable product metadata”
3) Design Templates: scalability rests upon standardisation and central design templates make things a lot easier.
A design template should include globally relevant & standardised tracking, dynamic sizing, a robust connection to the product catalogue, error validation and localisation handling
Getting it done:
4/10
tricky
1
week
Getting it right:
7/10
tricky
3
weeks
You need: front-end developer with ~3yrs experience & a recommendations-tool-specific developer with ~2yrs experience.
The hard part
- Nuances of the tool-specific pseudo-language and tool-specific debugging
- Building the template such that it works well for all products in all markets demands experience

Cross-device/ browser compatibility & tool-specific special characters

Outcome: best practice clean & highly functional product recommendations are worth >50% more than your typical entry-level design*. Design templates first capture this boost then facilitate rapid scale.
“clean & highly functional product recommendations are worth >50% more”
4) User Needs: what’s it all for?
There are hundreds of products we could throw at each page, but a good needs-first framework will cut this down to 2-3 fundamental needs and priority-ranked options within each.
Testing out these options will form the backbone of your first 6-12 month program.
Getting it done:
1/10
tricky
1
week
Getting it right:
4/10
tricky
3
weeks
You need: someone who’s done this a few times before to point you in the right direction and steer around potholes. Not something you need a coding background for.
The hard part
- Knowing the brand well enough to identify user needs, while also understanding the nuances of each algorithmic offering, off the back of a few year’s experience working around obstacles.

Outcome: cannibalisation can soar beyond 100%* where two recommendations serve the same purpose, distracting and confusing. Targeting user needs tailored to your audience protects from this risk & prevents unnecessarily bloated UX.
“cannibalisation can soar beyond 100%* where two recommendations serve the same purpose”
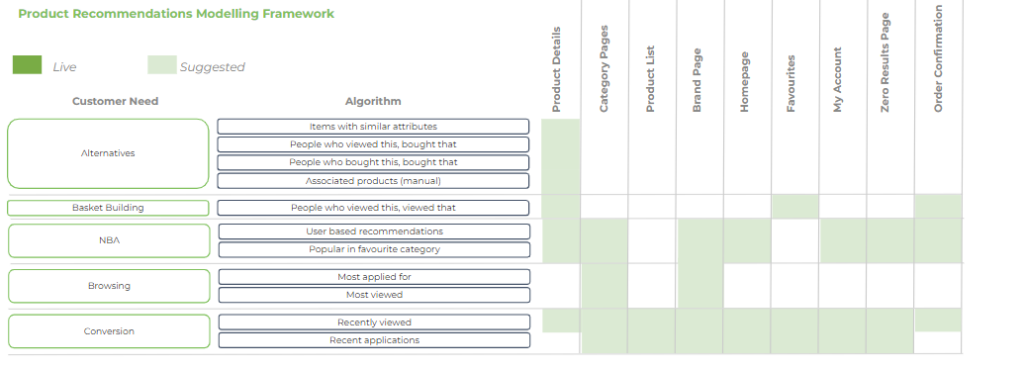
5) Product algorithms: the genius and really the whole point of product recommendations.
A good tool will include a few standard algorithms for each user need and will be built to accept custom models, as your program evolves.
Getting it done:
1/10
tricky
1
day (with a good tool)
Getting it right:
5/10
tricky
3
weeks (including custom criteria)
You need: a recommendations-tool-specialist developer with strong awareness of the product library & metadata, and a TMS developer for those custom models.
The hard part
- Understanding & leveraging the long list of configurations available within the more advanced tools, from configuring your data source to setting filters & backup rules to ensure every customer gets a useful & complete offering.
- At the simplest level this can be easy, with the challenge growing in line with complexity of business and maturity of program.

Outcome: Moving beyond default algorithms opens up the whole suite of user needs and facilitates hyper-personal offerings. Models built to incorporate recency, frequency & category of an entire order history are rarely seen and hyper-relevant, 3X as powerful in revenue terms as those based on an individual product ordered*
“Models built to incorporate recency, frequency & category of an entire order history on average 3x as powerful”
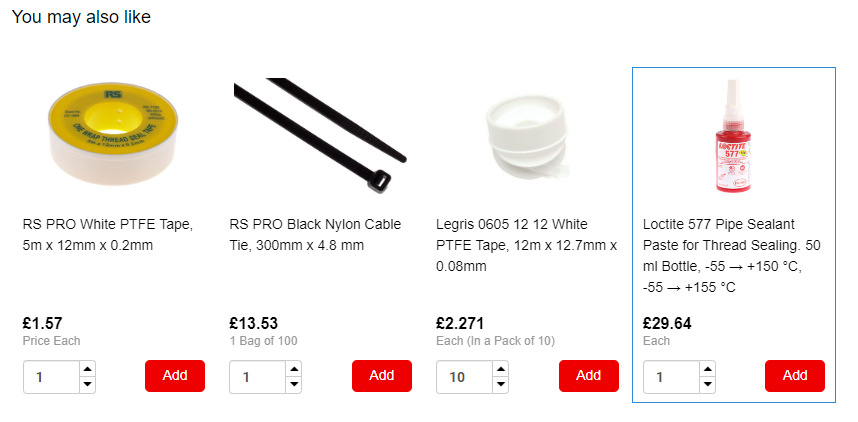
6) Audiences: identifying audiences before you need them is like remembering to pin down your tent, it’s a small job now for a big boost later.
The returns on straight-up optimisation will diminish over time and you then turn to audience-targeting to keep your foot down.
Similar to those user needs, audiences should start as an informed guess at the phases your customers go through, to be refined & leveraged as we learn.
Getting it done:
2/10
tricky
2
days
Getting it right:
5/10
tricky
3
weeks
You need: someone with experience building & testing lifecycle audiences to fast-track you through a few years’ learning, and an expert in your recommendations & analytics tools to bring this all to life.
The hard part
- Balancing the enormous opportunity of pinpoint personalisation with the inevitable increase in resource demand from maintaining far more distinct experiences. Again this is all about experience.
- Once you’ve seen programs crippled by admin and others failing to grasp anything, you get a feel for the optimal middleground.

Outcome: purchase cycle audience targeting triples revenue impact on av.* by hiding where a recommendation damages conversion, for example by distracting at a conversion-close moment.
“purchase cycle audience targeting triples revenue impact on average”
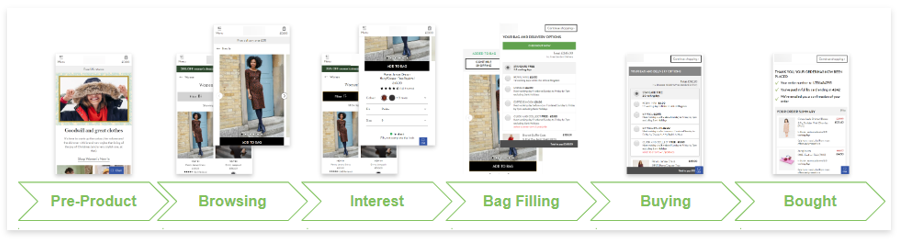
Your competitors are sucking in hoards of digital newbies and making 8 figures while you deliberate over the brand-fit and return on effort from intelligent product recommendations. Radical change has never been more achievable (see steps 1-3) and the digital maturity that’s 5 years away for the wider business can be leapt to in a matter of months within the uniquely insulated & mappable world of product recommendations (see steps 4-6). If you offer more than a handful of products, you need to get off the fence and catch up as fast as you can.
It might be that a quick conversation is all you need to get on your way. Message DMPG founder Steve for an open and frank conversation, free of cost and hyperbole.
*Parting Considerations (based on data)
- Digital spend still 32% above pre-pandemic levels
- See ONS publication Retail sales, Great Britain: March 2022 > “Retail sales, selected sectors”. This 32% figure is non-store Dec ‘21 – Feb ‘22 revenue vs non-store Dec ‘19 – Feb ‘20 revenue. December – February chosen to enable YoY pre-pandemic comparison.
- Statement: “millions drawn online during the pandemic”
- June 2020 PYMNTS study finds that share of US consumers buying online rose from 29.2% to 35.7%. This 6.5% of the USA’s ~258.3M adults (ref) equates to 16.8M new to online. While it is likely that not all US adults are classed as “consumers” here, this figure easily justifies use of the term “millions”
- Statement: best practice clean & highly functional product recommendations are worth >50% more than your typical entry-level design*
- One DMPG client revised their product recommendations module design, introducing more product data, the ability to add to cart, and going for a cleaner aesthetic. This change led to a +56% increase in engagement, and similar with other clients have had comparable results.
- Statement: This is a big part of what you’re paying for and by getting it right, you’re ready to scale the 2.5% – 4% revenue growth* that will come later
- Amongst those DMPG clients operating product recommendations across the majority of the user journey, 2.5-4% of all digital revenue is typically attributed to product recommendations
- Statement: Models built to incorporate recency, frequency & category of an entire order history on average 3x as powerful
- DMPG client testing one recommendation combining most common category purchased with highest value product purchased, with revenue/ view 200% higher than a similar recommendation based only on the most recently purchased product
- Statement: DMPG clients make 10-30% of program revenue from recommendations dependent on ubiquitous & reliable product metadata
- Amongst those DMPG clients operating product recommendations across the majority of the user journey, 10-30% of revenue is attributed to experiences that would not be at all possible without product metadata that covers near-all products and which can be trusted.
- Statement: cannibalisation can soar beyond 100% where two recommendations serve the same purpose
- Source: One large DMPG client. Both category affinity & brand affinity recommendations existed on the same page serving the same user need. Hiding one of these led to a net increase in attributed revenue, so we can say that>100% of the attributed revenue from the 2nd recommendation was in fact cannibalised from the first.
- Statement: purchase cycle audience targeting triples revenue impact on average
- Source: One large DMPG client, analysis comparing annualised incremental revenue of recommendations program with equivalent figure where each recommendation is deactivated for all purchase phases where revenue/ unique visitor impact is negative